To produce an image, a camera relies on a lens to redirect light onto a sensor that converts the light into digital signals interpreted by a computer as color. Without a lens, the result is a blurry, pixelated image that is unrecognizable to the naked eye. However, within the pixilated photo is enough digital information to detect the object of the image if a computer program is trained to identify it.
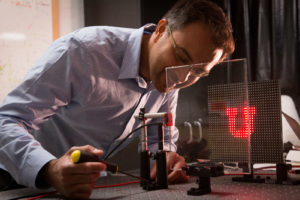
In 2018, Electrical and computer engineering (ECE) associate professor Rajesh Menon developed a lensless camera capable of doing just that. During a series of experiments, Menon and his team of researchers took a picture of the University of Utah’s “U” logo as well as a video of an animated stick figure, both displayed on an LED light board. The resulting image from the camera sensor, with help from a computer processor running the algorithm, was a recognizable but low-resolution image.
Menon has since designed two new algorithms with ECE undergraduate student Soren Nelson capable of interpreting more complex information and producing higher-quality images. In eliminating all optics and leaving only the image sensor with a thickness of less than 1mm, they have created the world’s thinnest possible camera. The two detailed their innovation in the paper, “Bijective-constrained cycle-consistent deep learning for optics-free imaging and classification,” recently published in the high impact journal Optica. The paper resulted from Nelson’s undergraduate research project, which he completed before graduating in Fall 2020.
While the first algorithm predicts a forward model, interpreting the light that reflects from an object to the camera’s sensor, the other does the opposite. Each algorithm must then verify its prediction matches the others. The deep neural network architectures created by Nelson and Menon run simultaneously and can intrinsically learn the physics of the imaging process. Not only is their work the first to apply this approach, but it also enables the interpretation of structurally diverse images, while methods utilizing only a forward model have been unsuccessful in doing the same.
Menon envisions numerous potential applications for their work including, home windows that double as security cameras, improved car cameras for sensing objects on the road and monitoring oil and gas pipelines. He sees potential for its use in enhancing privacy such as by replacing current fingerprint and facial recognition techniques with an optics-free photo that would be meaningless to another human if stolen. Menon also hopes to explore possible applications in other wavelengths like infrared, x-ray, and more.
Students interested in this work can attend Menon’s project-based class, 5960/6960 Computational Photography, where they will receive hands-on experience building cameras and developing algorithms. For more information on this work, visit Menon’s Lab.